How to Stop Deep Fake Impersonation with Advanced Identity Verification
The digital world is a double-edged sword. It brings us convenience and connection, but also opens doors to new forms of deceit. Deep fakes, hyper-realistic AI-generated videos mimicking real people, are a prime example. This technology, once confined to the realm of science fiction, is now readily accessible and poses a significant threat to online security, particularly in the context of identity verification.
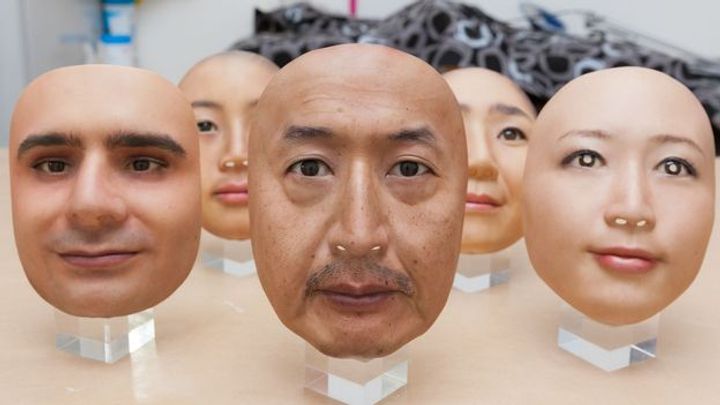
Introduction
In recent years, deepfake technology has emerged as a disruptive force, challenging traditional methods of identity verification. As artificial intelligence (AI) continues to advance, the ability to create highly convincing synthetic media has become increasingly accessible. Deepfakes pose a significant threat to individuals and businesses alike, as they can be used to impersonate identities, spread misinformation, and facilitate fraudulent activities.
In this comprehensive guide, we will explore the world of deepfakes, their impact on identity verification processes, and the cutting-edge solutions that can help mitigate the risks associated with this emerging technology. We will delve into the mechanics of deepfakes, their potential consequences, and the strategies businesses can adopt to safeguard their operations against deepfake-induced fraud.
Understanding Deepfakes
What are Deepfakes?
Deepfakes, a portmanteau of "deep learning" and "fake," refer to synthetic media created or manipulated by AI-powered deep learning techniques. These techniques analyze and learn from vast datasets of real media, enabling the generation of highly realistic audio, video, or image content that can convincingly impersonate individuals.
How are Deepfakes Created?
The creation of deepfakes typically involves two main components: a generator and a discriminator. The generator is responsible for producing synthetic media, while the discriminator evaluates the generated content against real data, providing feedback to refine the output. This iterative process allows the generator to improve its ability to create convincing fakes.
One popular approach to creating deepfakes is through Generative Adversarial Networks (GANs). GANs consist of two neural networks – a generator and a discriminator – that compete against each other. The generator attempts to produce synthetic data that can fool the discriminator, while the discriminator tries to distinguish between real and fake data. Over time, this adversarial training process results in the generator becoming increasingly proficient at creating realistic deepfakes.
Types of Deepfakes
Deepfakes can take various forms, including:
- Face Swaps: Superimposing a source face onto a target individual's body or head, creating a convincing impression that the target person is speaking or acting.
- Fully Generated Images: Leveraging generative models trained on vast datasets to create highly realistic images of faces that do not exist in reality.
- Lip-Sync Videos: Manipulating the lip movements of an individual in a video, often accompanied by a deepfaked voice, to create the illusion of them saying words they never uttered.
The Impact of Deepfakes on Identity Verification
Challenges to Facial Recognition Systems
Facial recognition technology is a crucial component of digital identity verification processes. However, the advent of deepfakes has introduced several challenges to this domain. Sophisticated deepfakes can deceive facial recognition systems, leading to potential identity fraud and security breaches.
Economic Implications of Deepfake Fraud
The economic toll of deepfake fraud is substantial and growing. Recent statistics reveal that a significant portion of companies has already experienced deepfake fraud attempts, with losses reaching staggering amounts. As deepfake technology continues to advance, the threat of financial losses due to identity fraud is likely to escalate.
Combating Deepfake Impersonation
Liveness Biometric Verification
One effective way to counter deepfakes is to leverage liveness biometric verification technology. This process involves verifying that the individual attempting to gain access or authenticate their identity is a live, present human being, rather than a synthetic or pre-recorded deepfake. Liveness detection techniques can include prompting the user to perform specific actions, such as blinking, nodding, or reciting a randomly generated phrase.
Document Verification
In addition to biometric verification, document verification plays a crucial role in combating deepfake impersonation. This process involves verifying the authenticity of identity documents, such as passports or driver's licenses, by analyzing security features and cross-checking against official databases. By combining biometric and document verification, businesses can establish a more robust identity verification process, reducing the risk of deepfake-induced fraud.
Advanced Deepfake Detection Techniques
To stay ahead of the ever-evolving deepfake threat, businesses and researchers are developing advanced detection techniques. These techniques leverage a combination of machine learning algorithms, image and video analysis, and metadata analysis to identify artifacts, inconsistencies, and patterns indicative of deepfake content. Some of the techniques employed include:
- Facial Motion Analysis: Detecting unnatural facial animations and suspicious patterns that may indicate manipulation.
- Audio Analysis: Identifying discrepancies in lip synchronization, audio quality, and frequency that could signify deepfake audio manipulation.
- Texture Analysis: Analyzing skin and hair textures for potential signs of manipulation or synthetic generation.
- Metadata Analysis: Verifying the authenticity of video metadata, such as creation date, time, and geographic location, to detect potential inconsistencies.
- Error Level Analysis: Analyzing the compression levels and frequency patterns of images or videos to detect potential face point mismatches or other artifacts.
Implementing a Robust Identity Verification Strategy
To effectively mitigate the risks posed by deepfakes, businesses must adopt a comprehensive identity verification strategy that incorporates multiple layers of security.
Multi-Factor Authentication
Implementing multi-factor authentication (MFA) can significantly enhance the security of identity verification processes. MFA combines multiple authentication factors, such as biometrics (e.g., facial recognition or fingerprint scanning), knowledge factors (e.g., one-time passwords or security questions), and possession factors (e.g., mobile devices or hardware tokens). By requiring multiple authentication factors, businesses can reduce the likelihood of successful deepfake impersonation attempts.
Continuous Monitoring and Adaptation
As deepfake technology continues to evolve, it is crucial for businesses to continuously monitor and adapt their identity verification systems. This may involve regularly updating anti-spoofing models, incorporating new detection techniques, and staying informed about the latest deepfake trends and threats. Proactive monitoring and adaptation can help businesses stay ahead of potential vulnerabilities and maintain the effectiveness of their identity verification processes.
User Education and Awareness
In addition to technological measures, businesses should prioritize user education and awareness initiatives. Educating users about the risks associated with deepfakes, teaching them how to identify potential deepfake content, and encouraging them to report suspicious activities can significantly enhance the overall security posture of an organization.
The Future of Identity Verification
As the battle against deepfakes intensifies, the identity verification landscape is poised to undergo significant transformations. The integration of cutting-edge technologies, such as blockchain, quantum computing, and advanced biometrics, could pave the way for more secure and tamper-proof identity verification systems.
Additionally, the development of decentralized identity solutions, where individuals have greater control over their personal data and identities, may emerge as a viable alternative to traditional centralized identity management systems.
Conclusion
Deepfakes pose a significant challenge to traditional identity verification processes, with potential far-reaching consequences for individuals and businesses alike. However, by embracing advanced technologies, implementing robust multi-layered security measures, and fostering a culture of continuous adaptation and user awareness, organizations can effectively mitigate the risks associated with deepfake impersonation.
As the digital landscape continues to evolve, the importance of secure and reliable identity verification will only increase. By staying vigilant, investing in cutting-edge solutions, and collaborating with industry experts and researchers, businesses can stay ahead of the deepfake threat and safeguard the integrity of their operations.